|
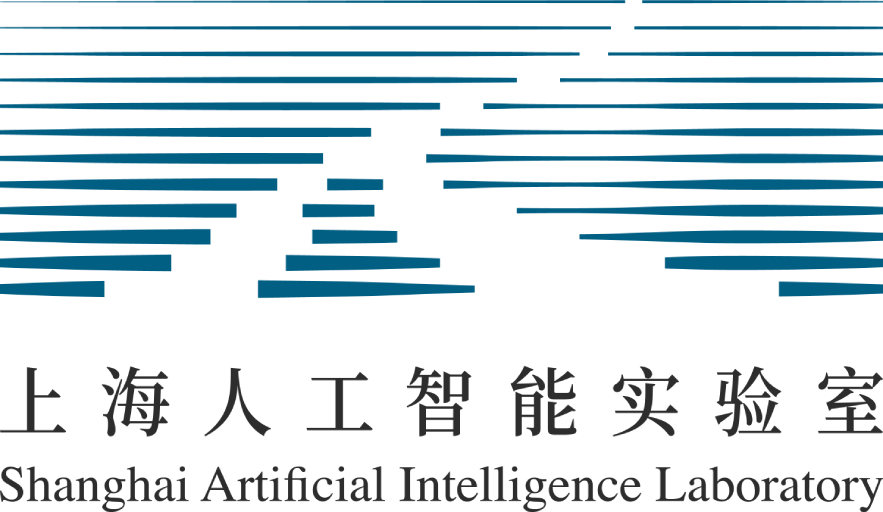 |
Hongyang Li |
Opening Remarks |
|
|
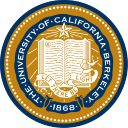 |
Sergey Levine UC Berkeley, USA |
Robotic Foundation Models |
|
|
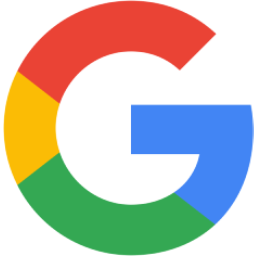 |
Sherry Yang Google DeepMind, USA |
Foundation Models as Real-World Simulators |
|
|
|
Coffee Break |
☕️ |
|
|
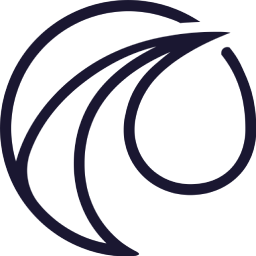 |
Alex Kendall Wayve, UK |
Building Embodied AI to be Safe and Scalable |
|
|
 |
Autonomous Grand Challenge Part Ⅰ |
- End-to-End Driving at Scale
- Introduction (5 min, Igor Gilitschenski)
- Innovation Award & Outstanding Champion (10 min)
- Predictive World Model
- Introduction (5 min, Zetong Yang)
- Innovation Award & Honorable Runner-up (10 min)
- Multi-View 3D Visual Grounding
- Introduction (5 min, Tai Wang)
- Innovation Award & Outstanding Champion (10 min)
- Occupancy and Flow
- Introduction (5 min, Jiazhi Yang)
- Outstanding Champion (10 min)
|
|
|
|
Lunch Break |
Poster Session of Autonomous Grand Challenge |
|
|
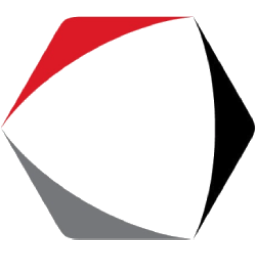 |
Rares Ambrus Toyota Research Institute, USA |
Visual Foundation Models for Embodied Applications |
|
|
 |
Autonomous Grand Challenge Part Ⅱ |
- Mapless Driving
- Introduction (5 min, Huijie Wang)
- Innovation Award & Outstanding Champion (10 min)
- Driving with Language
- Introduction (5 min, Chonghao Sima)
- 1st Place (5 min)
- 2nd Place (5 min)
- CARLA Autonomous Driving Challenge
- Introduction (5 min, Matt Rowe)
- Innovation Award & Outstanding Champion (10 min)
|
|
|
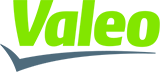 |
Andrei Bursuc Valeo, France |
Foundation Models in the Automotive Industry |
|
|
|
Coffee Break |
☕️ |
|
|
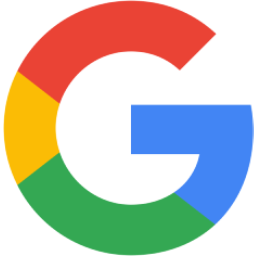 |
Ted Xiao Google DeepMind, USA |
What's Missing for Robotics-first Foundation Models? |
|
|
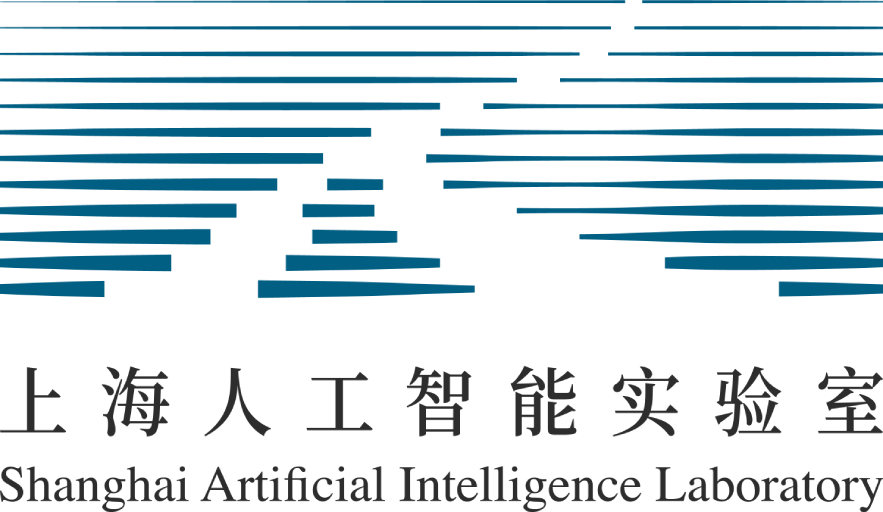 |
Li Chen Shanghai AI Lab, China |
Visual World Models as Foundation Models for Autonomous Systems |
|
|
|
Panel Host: Anthony Hu |
Challenges in Building Foundations Models for Embodied AI
Panelist: Andrei Bursuc, Alex Kendall, Hongyang Li, Christos Sakaridis, Ted Xiao
|
|