Leaderboard (Server remains active)
# Participating Teams: 152
# Countries and Regions: 14
# Submissions: 978
* Not involed in the final official ranking.
† Selected submission from previous scores, not the highest one.
Innovation Award goes to ADLM for
Team ADLM uses a spatially unified BEV feature and aligns it with an LLM to help the LLM comprehend spatial relationship information. The whole pipeline is in an end-to-end fashion.
Award
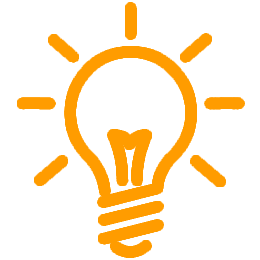 | Innovation Award | USD 1,000 |
 | Outstanding Champion | USD 500 |
Citation
Please consider citing our work if the challenge helps your research with the following BibTex:
@article{sima2023drivelm,
title={DriveLM: Driving with Graph Visual Question Answering},
author={Sima, Chonghao and Renz, Katrin and Chitta, Kashyap and Chen, Li and Zhang, Hanxue and Xie, Chengen and Luo, Ping and Geiger, Andreas and Li, Hongyang},
journal={arXiv preprint arXiv:2312.14150},
year={2023}
}
@misc{contributors2023drivelmrepo,
title={DriveLM: Driving with Graph Visual Question Answering},
author={DriveLM contributors},
howpublished={\url{https://github.com/OpenDriveLab/DriveLM}},
year={2023}
}
@article{zhou2024embodied,
title={Embodied Understanding of Driving Scenarios},
author={Zhou, Yunsong and Huang, Linyan and Bu, Qingwen and Zeng, Jia and Li, Tianyu and Qiu, Hang and Zhu, Hongzi and Guo, Minyi and Qiao, Yu and Li, Hongyang},
journal={arXiv preprint arXiv:2403.04593},
year={2024}
}
Contact
Task Description
Incorporating the language modality, this task connects Vision Language Models (VLMs) and autonomous driving systems. The model will introduce the reasoning ability of LLMs to make decisions, and pursue generalizable and explainable driving behavior. Given multi-view images as inputs, models are required to answer questions covering various aspects of driving.
Details and test server are available at Hugging Face.
Related Literature